Visualization - choropleth-mapbox + plotly + python
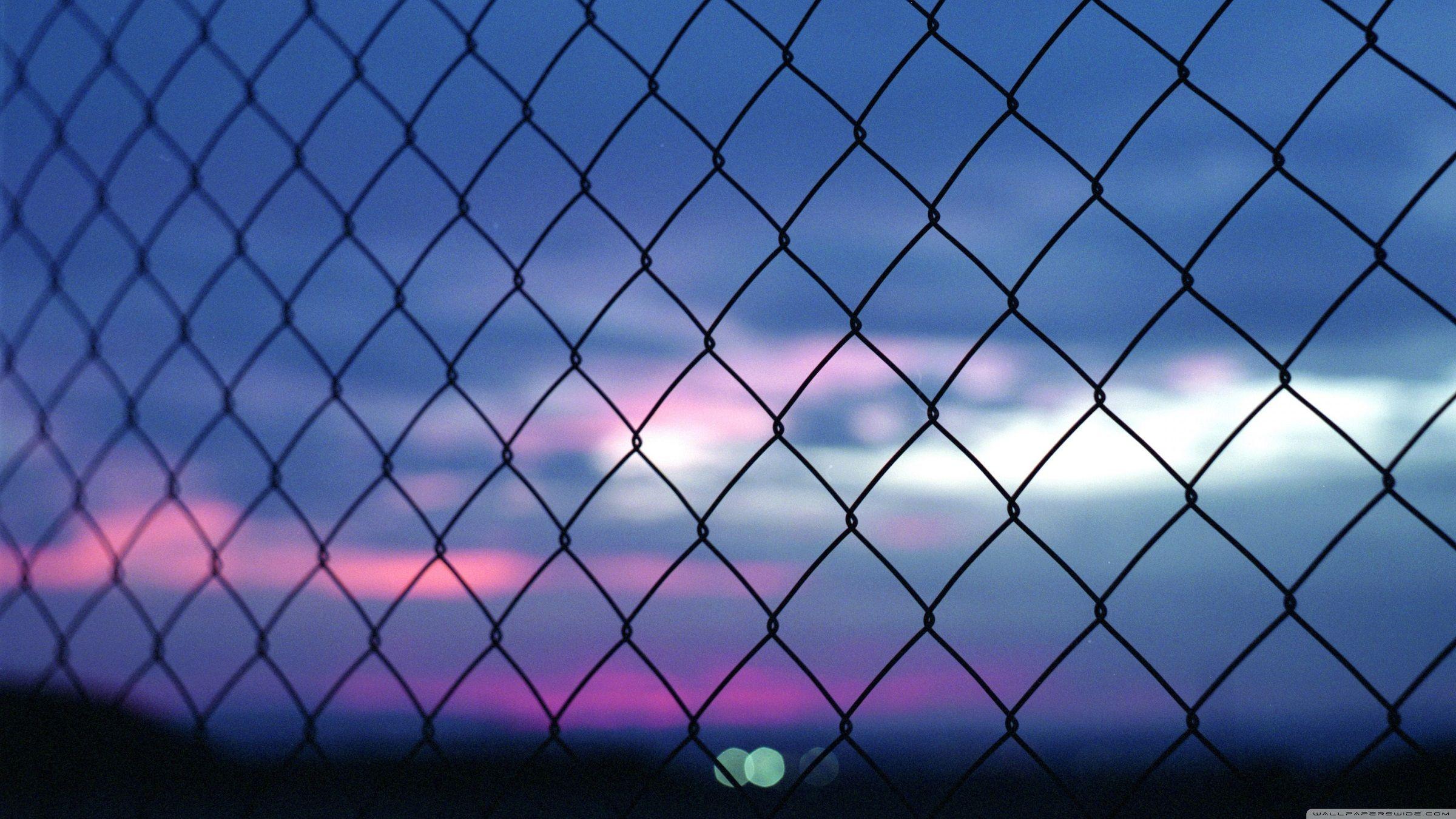
This post will introduce how to visualize the continuous variable on the map with the use of choropleth_mapbox, a mighty tool to realize animation.
Table of Contents
- Step 1. import all needed packages
- Step 2. import json file from existing file
- Step 3. make the json file smaller
- Step 4. Visualization through choropleth_mapbox
- Step 5. Export the image as local html
- Step 6. Save the image on plotly account
Step 1. import all needed packages
1 | import numpy as np |
Step 2. import json file from existing file
The .json file could be converted from shp. file. I convert it directly by using QGIS.
More conveniently, you can use package shapefile in python to finish the conversion. Check reference for more detail.
1 | with open('Simplify_GeoJSON_CHNcity.json') as f: |
1 | # examine the fearue of the json file |
{‘type’: ‘Feature’,
‘geometry’: {‘type’: ‘Polygon’,
‘coordinates’: [[[116.12962341, 29.82485008],
[116.27577972, 29.79137039],
[116.4651413, 29.90048981],
[116.54703522, 29.9179039],
[116.58567047, 30.05631828],…
You could find out that the coordinates is in 8 decimal places, which will inflate the size of the map. Therefore, we need the following step.
Step 3. make the json file smaller
First, check If you have large GeoJSON files, such as over 20 or 30 MB. The map upload tends to be slow. And normally could not be uploaded to plotly account.
The easiest way is to use https://mapshaper.org/ to simplify the json file, to make the json file better less than 3MB. You would not lose necessary information because we here care about various coloration across regions.
When you have already finish above two steps, your json file is already small enough. To make it even smaller, run following code to shrink it 10% or so.
1 | #Round off the locations to 2 decimal places (about 1.1 km accuracy) |
array([116.13, 29.82])
Step 4. Visualization through choropleth_mapbox
I have already prepared the df_EC for use, detail cleaning process is omitted here.
We need to ensure that the dataframe df_EC have the same location “id” as geojson file.
Please check the the official websie for setting the parrrameters: https://plotly.github.io/plotly.py-docs/generated/plotly.express.choropleth_mapbox.html
1 | fig_Heterogeneity = px.choropleth_mapbox(df_EC, |
In the default browser, we get the following image, indicating the environmental complexity across China.
Step 5. Export the image as local html
You might want it.. Even though I don’t think it’s that useful
1 | import plotly.io as pio |
Step 6. Save the image on plotly account
The url in the plotly website can help you disseminate your work. The url can also be used in ppt with animation. I will introduce the embeddment of it in my future blog.
You need to first go the this website for registering account. https://chart-studio.plotly.com/feed/#/
Installation of Chart Studio in python: https://plotly.com/python/getting-started-with-chart-studio/
Then upload the image to your account
1 | py.plot(fig_Heterogeneity, filename = 'Environmental Heterogeneity', auto_open=True) |
It uploads the image online including the data on your plotly account.
If returned with the following error!! Go back to step 3 to shrink the size.
“This file is too big! Your current subscription is limited to 524.288 KB uploads. For more information, please visit: https://plotly.com/get-pricing/."
Cheers!🥳
The following image depicts the environmental dispersion across China. Same steps above can get you this beautiful visualization.
References: